The Blind Men and the Elephant
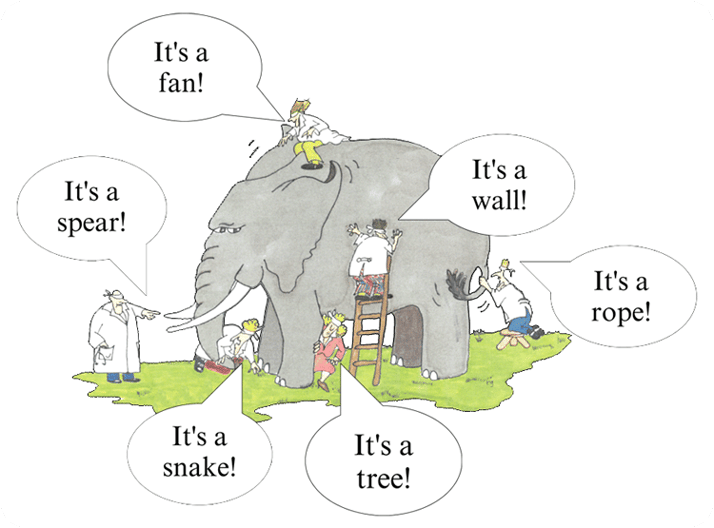
There is a parable from India about six men who had heard of, but had never seen, an elephant. One day, an elephant appeared, and each man approached to investigate. The first one, touching his side, claimed, ‘It’s a wall!’ the second, holding a leg, scoffed, saying, ‘it’s a tree’! And so on. The men sat and argued all day, doing nothing.
Sometimes people who can see act just as blindly.
This story is a classic example of a bottom-up approach - deciding a macro-level question with micro-level information.
How does this translate to manufacturing?
Consider a typical enterprise manufacturer:
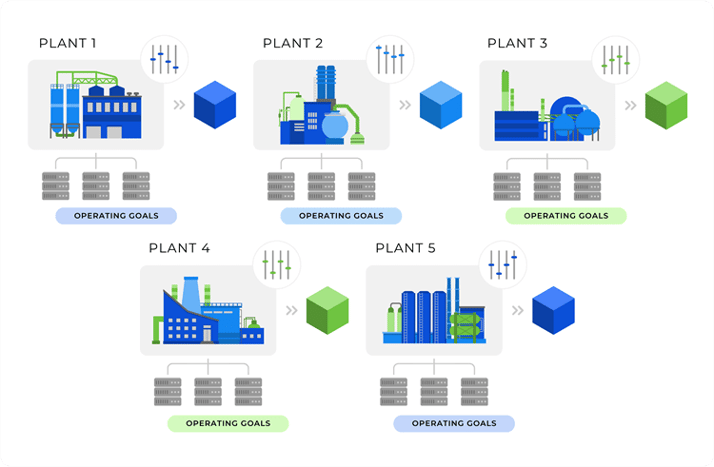
Think of any individual as one of the characters in the story. Instead of an elephant, they’re looking at how to deliver a goal limited by what they know, what they can see, and where they are looking. Nothing is wrong with what they’re doing, but in looking ‘small’, they miss the big picture and, thus, they miss the optimum way to meet the goal.
Let’s take a different look at our elephant:
This is a top-down approach - one that gathers information from all sources and presents it to everyone involved to come to a common understanding of the situation, what’s causing it, and the most likely candidates to address it. AI is a crucial element in turbocharging this critical process.
Elements of an AI-Assisted Top-Down Approach
Baselining: Getting a complete picture of your manufacturing enterprises using all data sources you have. This creates a picture of how things are now.
How AI helps: AI can ‘fill in the blanks’ of missing data, or enrich the data with analytically created insights, and contextualize the data so that a global enterprise with variability in units of measure, time slices, and levels of automation can be seen together.
Anomaly Detection: Shows you where your current processes are varying - within themselves, and from your best run processes. This step is the beginning of solving the macro problem by identifying where your best bets are for meeting your goals.
How AI helps: It can distinguish between correlation and causation, can apply the best analytics approach depending on the data it sees, and find hidden relationships between visually separate processes or parameters. It identifies a contextualized ‘best’ / golden batch / optimal operating conditions. Learns continuously with data adjustments and as new data comes in.
Root Cause Analysis: This allows you to drill down into likely causes of variation, eliminate factors you can't take action on (for example, those requiring capital expenditure), and ID those that are actionable.
How AI helps: Trains and retrains performance models in support of an interactive process where an actionable list of insights is created.
Operationalization: There is limited business impact before an insight is put into operation - where business or production processes change, where personnel are trained, where alerts go to operators who can action them, or to SCADA systems where they can be autonomously managed. KPI changes are the result of the top-down process, not necessarily the driver of a Top Down Process.
Now does this mean there is no place for bottom-up work? No - but bottom-up is the right choice only when you do not see like process, equipment, or product across your enterprise, and it can be concluded that a macro view is not beneficial.
I mentioned above that an AI-assisted top-down process learns. Without this learning, iterating, and updating, you’ve built a one-off solution that cannot respond to ongoing reality… and things change every day - weather, raw material quality, operator skill… and that is the ultimate value add to AI-assisted top-down approaches. They live, learn, and help you improve without having to start again from scratch.
Tags:
Blog, Digital Twin, Quality, Digital Thread, IIoT, AI, Contextualization, Visualization, Operationalize, Efficiency, KPIs, Manufacturing, Operational Metrics, Innovation, Energy Cost Reduction, Operational Costs
August 1, 2023
Sheila’s management philosophy produces results. By enforcing “just the right” amount of infrastructure, she enables teams to work towards the ultimate, shared goal of the highest quality customer experience.
She’s a proud graduate of Boston College (Business and Computer Science) and Babson (MBA 2019), a native New Yorker, and a fierce advocate for women in tech.